Check this out:
UltiHash is a gamechanger for LLM creation
As datasets used to train LLMs get ever larger, UltiHash is a genuinely sustainable storage solution
ultihash.io/blog/
ultihash-is-a-gamechanger-for-llm-creation
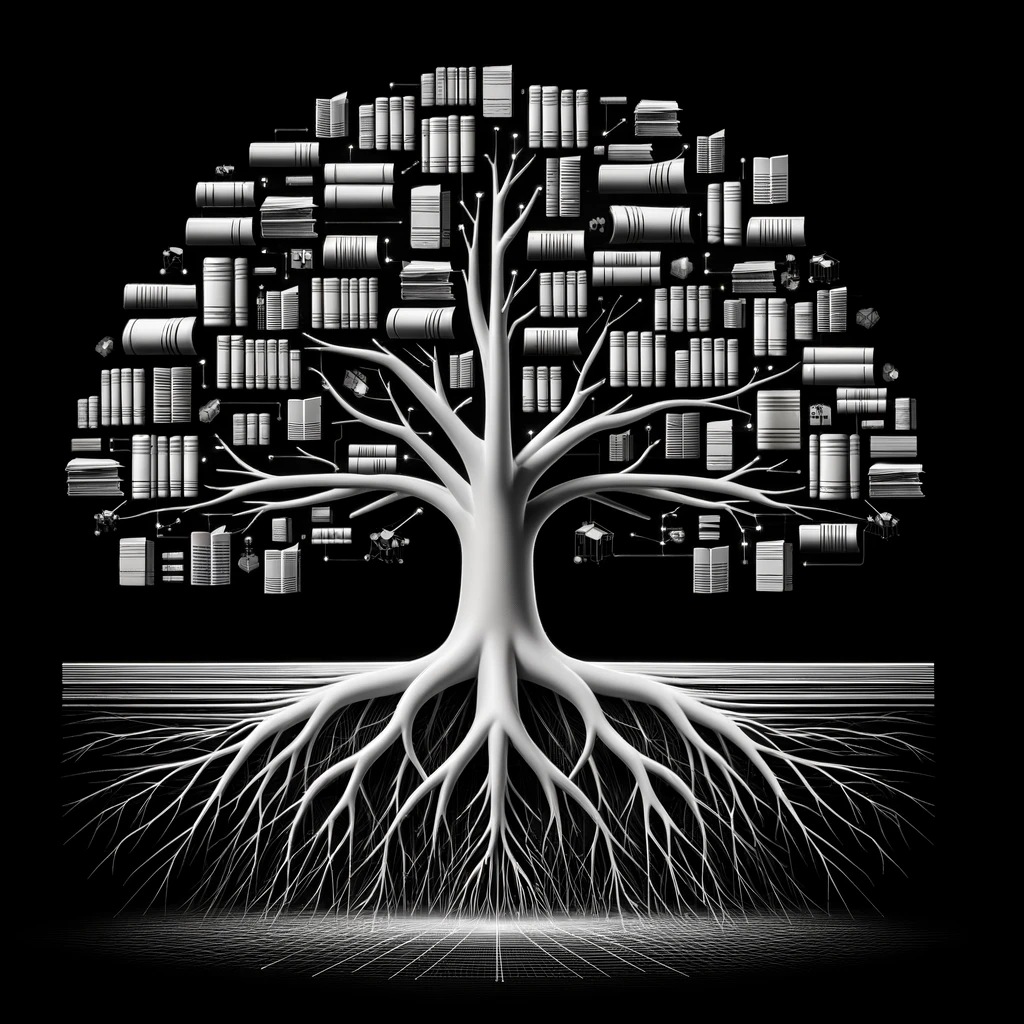
In the rapidly-evolving world of AI, LLMs are making huge waves, causing us to rethink the possibilities in a whole range of industries. But their immense sizes and complex structures present unique storage challenges that traditional methods struggle to address. Enter UltiHash: a next-generation storage platform designed to address these challenges head-on.
Two decades ago, in 2004, Google shook the data world with the introduction of MapReduce, which made parallel processing of large quantities of data within a reasonable period of time possible. This approach set off a cascade of different products stemming from that core technology; the whole Hadoop ecosystem is a shining example here. It also catalysed the rise of distributed file systems, like HDFS or MapR.
Today, we are witnessing another seismic moment: the advent of the Large Language Model (LLM).
Developing these models requires training on datasets that are quickly growing into the hundreds of terabytes, demanding storage solutions that not only accommodate their size but also facilitate efficient access and processing.
The challenge intensifies all the more when we consider the exponential growth of these models in just a short period of time. As the market seeks solutions to ever more complicated tasks, ever more sophisticated LLMs are required - which, in turn, require ever more advanced training and fine-tuning. What’s more, these demands are happening within increasingly short timeframes. For context: in March 2022, OpenAI’s GPT 3.5 released with a reported 175 billion parameters; just one short year later, GPT 4 released with a cool trillion parameters - almost six times as many. All these expanding numbers add up to a problem: as LLMs and their training datasets grow, so does the complexity of managing this data.
We’re developing a groundbreaking approach to storage that could transform the efficiency, scalability and sustainability of LLM training and creation. Our high-performance object storage layer is designed to handle the massive data sizes of LLM training datasets while maintaining optimal performance on read.
So how do we do this? We employ advanced deduplication techniques that allow us to significantly reduce the storage footprint of large datasets like LLM training corpuses. Files and folders are analyzed at the byte level, and dynamically split into fragments of different sizes. All repeated fragments in the dataset are losslessly deduplicated to save space, leaving only unique fragments. If a fragment has been stored in the cluster before, it is matched; brand-new fragments are added as normal.
This innovative approach deduplicates not just on the file or object level, but across entire datasets, making our technology fundamentally different from standard compression. Thanks to this technique, UltiHash can even reduce the size of already-compressed data. And we can do all this without compromising the all-important speed of access.
UltiHash is also built from the ground up for scalability, in two key ways.
The first - more conventional - way is automatically increasing the hardware resources employed by the system depending on the load. If more storage space is needed, UltiHash automatically adds more data nodes. Similarly, if there is a spike in request load, UltiHash will elastically spin up new resources to keep up with the required workoad.
The second way is more unique to the way UltiHash stores data. Exponential growth is no problem for UltiHash; in fact, rapidly expanding data volumes are where UltiHash excels: the more data in a dataset, the more opportunities for deduplication there are. As LLMs and their associated datasets grow in complexity and size, it will become more and more efficient to use a solution like UltiHash.
This kind of fundamental scalability will allow businesses to leverage the full potential of LLMs - even as they grow ever more massive - without being hindered by infrastructure limitations.
Working together, these two fundamental pillars of scalability will allow our customers to leverage the full potential of LLMs - even as they grow ever more massive - without being hindered by infrastructure limitations.
When developing an LLM, quick access and loading of data is pivotal. A core part of this is deep integration with customer’s existing infrastructure, leveraging the efficiency of existing workflows and obviating the need for lengthy and expensive restructuring.
That’s why a guiding principle of UltiHash's design philosophy has been seamless integration with, for example, querying engines like Apache Trino, Flink and Spark. In fact, we currently support applications that use S3-compatible APIs, and are working on implementing additional APIs to extend UltiHash’s compatiblity with an even wider range of frameworks.
As AI continues to advance, it’s clear that the storage needs of LLMs will only grow more significant - particularly when it comes to training data. We want to evolve alongside these changes, ensuring that businesses can harness the full power of LLMs without being constrained by conventional storage limitations. UltiHash is being built for a future where storage for advanced technologies like LLMs need to be highly efficient at storing large data volumes while remaining highly performant.
By offering a solution that combines scalability, efficiency, and innovative data management, UltiHash is leading the charge in transforming how we store and access the vast amounts of data needed to train these advanced AI models. As we continue to explore the frontiers of AI, UltiHash stands ready to support the journey, ensuring sustainability and performance will always remain a fundamental in machine learning.
In the rapidly-evolving world of AI, LLMs are making huge waves, causing us to rethink the possibilities in a whole range of industries. But their immense sizes and complex structures present unique storage challenges that traditional methods struggle to address. Enter UltiHash: a next-generation storage platform designed to address these challenges head-on.
Two decades ago, in 2004, Google shook the data world with the introduction of MapReduce, which made parallel processing of large quantities of data within a reasonable period of time possible. This approach set off a cascade of different products stemming from that core technology; the whole Hadoop ecosystem is a shining example here. It also catalysed the rise of distributed file systems, like HDFS or MapR.
Today, we are witnessing another seismic moment: the advent of the Large Language Model (LLM).
Developing these models requires training on datasets that are quickly growing into the hundreds of terabytes, demanding storage solutions that not only accommodate their size but also facilitate efficient access and processing.
The challenge intensifies all the more when we consider the exponential growth of these models in just a short period of time. As the market seeks solutions to ever more complicated tasks, ever more sophisticated LLMs are required - which, in turn, require ever more advanced training and fine-tuning. What’s more, these demands are happening within increasingly short timeframes. For context: in March 2022, OpenAI’s GPT 3.5 released with a reported 175 billion parameters; just one short year later, GPT 4 released with a cool trillion parameters - almost six times as many. All these expanding numbers add up to a problem: as LLMs and their training datasets grow, so does the complexity of managing this data.
We’re developing a groundbreaking approach to storage that could transform the efficiency, scalability and sustainability of LLM training and creation. Our high-performance object storage layer is designed to handle the massive data sizes of LLM training datasets while maintaining optimal performance on read.
So how do we do this? We employ advanced deduplication techniques that allow us to significantly reduce the storage footprint of large datasets like LLM training corpuses. Files and folders are analyzed at the byte level, and dynamically split into fragments of different sizes. All repeated fragments in the dataset are losslessly deduplicated to save space, leaving only unique fragments. If a fragment has been stored in the cluster before, it is matched; brand-new fragments are added as normal.
This innovative approach deduplicates not just on the file or object level, but across entire datasets, making our technology fundamentally different from standard compression. Thanks to this technique, UltiHash can even reduce the size of already-compressed data. And we can do all this without compromising the all-important speed of access.
UltiHash is also built from the ground up for scalability, in two key ways.
The first - more conventional - way is automatically increasing the hardware resources employed by the system depending on the load. If more storage space is needed, UltiHash automatically adds more data nodes. Similarly, if there is a spike in request load, UltiHash will elastically spin up new resources to keep up with the required workoad.
The second way is more unique to the way UltiHash stores data. Exponential growth is no problem for UltiHash; in fact, rapidly expanding data volumes are where UltiHash excels: the more data in a dataset, the more opportunities for deduplication there are. As LLMs and their associated datasets grow in complexity and size, it will become more and more efficient to use a solution like UltiHash.
This kind of fundamental scalability will allow businesses to leverage the full potential of LLMs - even as they grow ever more massive - without being hindered by infrastructure limitations.
Working together, these two fundamental pillars of scalability will allow our customers to leverage the full potential of LLMs - even as they grow ever more massive - without being hindered by infrastructure limitations.
When developing an LLM, quick access and loading of data is pivotal. A core part of this is deep integration with customer’s existing infrastructure, leveraging the efficiency of existing workflows and obviating the need for lengthy and expensive restructuring.
That’s why a guiding principle of UltiHash's design philosophy has been seamless integration with, for example, querying engines like Apache Trino, Flink and Spark. In fact, we currently support applications that use S3-compatible APIs, and are working on implementing additional APIs to extend UltiHash’s compatiblity with an even wider range of frameworks.
As AI continues to advance, it’s clear that the storage needs of LLMs will only grow more significant - particularly when it comes to training data. We want to evolve alongside these changes, ensuring that businesses can harness the full power of LLMs without being constrained by conventional storage limitations. UltiHash is being built for a future where storage for advanced technologies like LLMs need to be highly efficient at storing large data volumes while remaining highly performant.
By offering a solution that combines scalability, efficiency, and innovative data management, UltiHash is leading the charge in transforming how we store and access the vast amounts of data needed to train these advanced AI models. As we continue to explore the frontiers of AI, UltiHash stands ready to support the journey, ensuring sustainability and performance will always remain a fundamental in machine learning.